Introduction
After getting my MSc and engineering degree in computer science and machine learning at the INSA of Rouen, I joined the CEDRIC at le CNAM in Paris to study Deep Learning and Computer Vision under the supervision of Nicolas Thome. I work directly with Visible Patient which develop a software for professionals in the medical field. Thanks to that partnership I get my PhD degree in decembre 2021 in deep learning applied to medical images. I'm very proud and happy to bring Artificial Intelligence in medical applications.
Activity
- 17/12/2021 : PhD defense at le CNAM
- 11/10/2021 : presentation of U-Transformer at the GdR ISIS day
- 27/09/2021 : oral presentation of U-Transformer at MLMI, MICCAI 2021
- 07/09/2021 : accepted paper 3D Spatial Priors for Semi-Supervised Organ Segmentation with Deep Convolutional Neural Networks at IJCARS
- 08/08/2021 : accepted paper U-Net Transformer: Self and Cross Attention for Medical Image Segmentation at MLMI MICCAI 2021
- 13/05/2021 : accepted paper Iterative Confidence Relabeling with Deep ConvNets for Organ Segmentation with Partial Labels at CMIG
- 10/04/2019 : presentation of SMILE at the GdR ISIS day [Slides]
Publications
2021
Olivier Petit.
Thesis
"Semantic Segmentation of 3D Medical Images with Deep Learning"
[PDF]
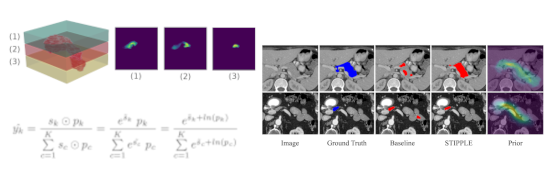
Olivier Petit, Nicolas Thome and Luc Soler.
"3D Spatial Priors for Semi-Supervised Organ Segmentation with Deep Convolutional Neural Networks"
IJCARS 2021 [PDF]
Purpose: Fully Convolutional neural Networks (FCNs) are the most popular models for medical image segmentation. However, they do not explicitly integrate spatial organ positions, which can be crucial for proper labeling in challenging contexts.
Methods: In this work, we propose a method that combines a model representing prior probabilities of an organ position in 3D with visual FCN predictions by means of a generalized prior-driven prediction function. The prior is also used in a self-labeling process to handle low-data regimes, in order to improve the quality of the pseudo-label selection.
Results: Experiments carried out on CT scans from the public TCIA pancreas segmentation dataset reveal that the resulting STIPPLE model can significantly increase performances compared to the FCN baseline, especially with few training images. We also show that STIPPLE outperforms state-of-the-art semi-supervised segmentation methods by leveraging the spatial prior information.
Conslusion: STIPPLE provides a segmentation method effective with few labeled examples, which is crucial in the medical domain. It offers an intuitive way to incorporate absolute position information by mimicking expert annotators.
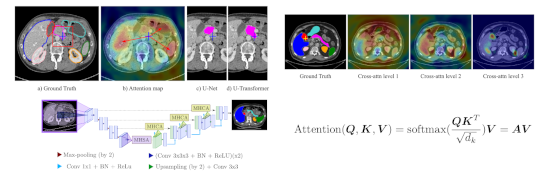
Olivier Petit, Nicolas Thome, Clement Rambour, Loic Themyr, Toby Collins and Luc Soler.
"U-Net Transformer: Self and Cross Attention for Medical Image Segmentation"
MICCAI 2021 workshop MLMI [PDF] [arXiv]
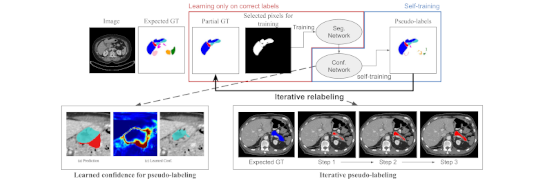
Olivier Petit, Nicolas Thome and Luc Soler.
"Iterative Confidence Relabeling with Deep ConvNets for Organ Segmentation with Partial Labels"
Computerized Medical Imaging and Graphics, 2021 [PDF]
2019
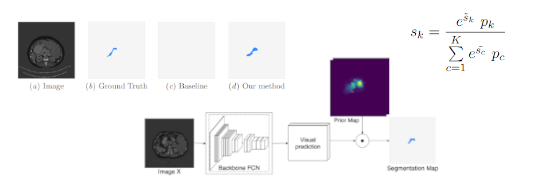
2018
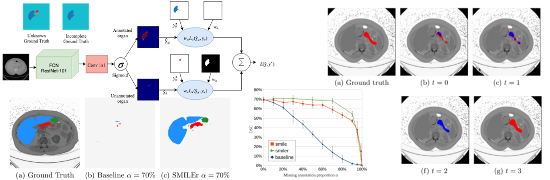
Olivier Petit, Nicolas Thome, Arnaud Charnoz, Alexandre Hostettler and Luc Soler.
"Handling Missing Annotations for Semantic Segmentation with Deep ConvNets."
MICCAI 2018 workshop DLMIA [PDF]